Unlocking the Power of Machine Learning for Email Spam Filtering
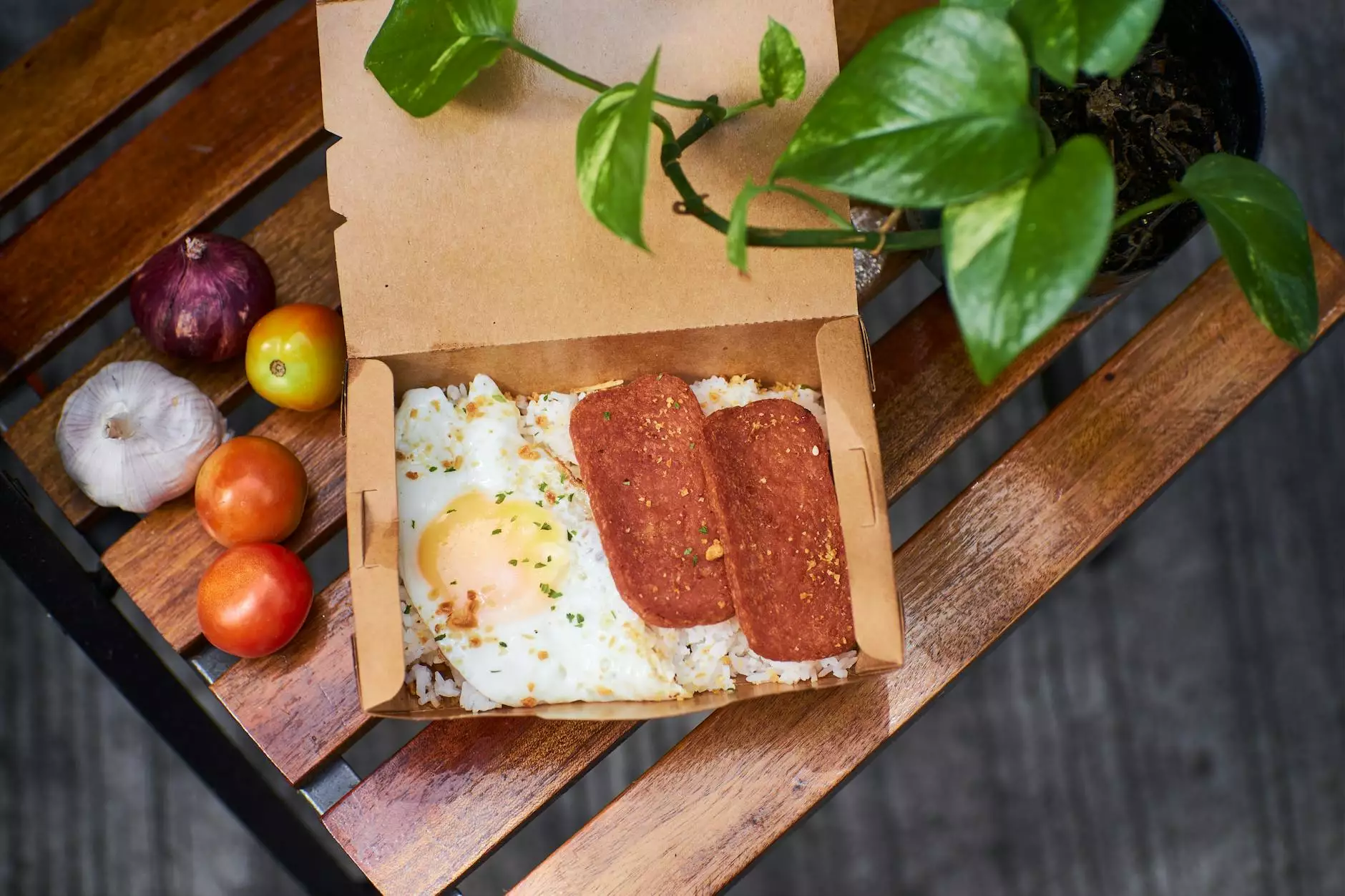
As businesses increasingly rely on digital communication, the threat of spam emails continues to grow. Organizations face significant challenges in managing their inboxes while ensuring that legitimate communications are not lost amidst the clutter of spam. This is where machine learning for email spam filtering comes into play, providing an innovative and effective solution.
The Evolution of Spam Filtering
Spam emails have undergone a significant evolution over the years, becoming more sophisticated and harder to detect. Traditional filtering methods relied on simple keyword matching and predefined rules, which often resulted in false positives (legitimate emails marked as spam) or false negatives (spam emails that were not detected). With the advent of machine learning, we now have a more dynamic approach to combat this issue.
What is Machine Learning?
Machine learning is a subset of artificial intelligence (AI) that enables systems to learn from data and improve over time without explicit programming. By using algorithms, machines can analyze vast amounts of data, identify patterns, and make predictions based on that analysis. This capability is particularly useful in filtering spam emails, as it allows for adaptive learning based on user behavior and changing spam tactics.
How Machine Learning Enhances Email Spam Filtering
When it comes to the application of machine learning for email spam filtering, several key mechanisms are employed:
- Data Collection: Machine learning models require extensive training data. Spam filtering systems collect email data, including marked spam and legitimate emails, to create a robust training dataset.
- Feature Extraction: Features, or characteristics of emails, are extracted for analysis. These features may include sender reputation, subject line analysis, email content, and historical user interactions.
- Model Training: Algorithms such as Naive Bayes, Support Vector Machines, and Neural Networks are used to train models. They learn to differentiate between spam and legitimate emails based on the extracted features.
- Real-Time Filtering: After training, the model is deployed to evaluate incoming emails in real time, providing instant categorization and protection against spam.
Benefits of Machine Learning in Spam Filtering
The implementation of machine learning for email spam filtering brings numerous advantages to businesses:
1. Enhanced Accuracy
Machine learning models continuously learn from new data, improving their accuracy in identifying spam messages. This reduces the likelihood of false positives and ensures that important emails are not misclassified.
2. Adaptive Learning
As spam techniques evolve, machine learning algorithms can adapt to new trends, ensuring that your spam filter remains effective against the latest tactics employed by spammers.
3. User Customization
Machine learning systems can be tailored to match individual user behaviors. By analyzing user interaction with emails, the system can learn preferences and provide personalized filtering.
4. Resource Efficiency
Automating the spam filtering process frees up valuable time for IT departments and employees, allowing them to focus on more critical tasks and boosting overall productivity.
Challenges in Implementing Machine Learning for Email Spam Filtering
Despite its many benefits, the implementation of machine learning for email spam filtering is not without its challenges:
- Data Quality: The effectiveness of machine learning algorithms significantly relies on the quality of data. Inaccurate or incomplete data can lead to inadequate model performance.
- Model Overfitting: If a model is too complex, it may perform well on training data but poorly on unseen data. Striking a balance between model complexity and performance is crucial.
- Privacy Concerns: The collection and analysis of email data raise privacy issues that must be addressed through compliance with regulations like GDPR.
Best Practices for Leveraging Machine Learning in Spam Filtering
To effectively utilize machine learning for email spam filtering, consider these best practices:
1. Continuous Training
Regularly update your models with new data to keep them relevant and improve accuracy. Adding fresh examples of spam and legitimate emails will help the model adapt to evolving patterns.
2. Implement Feedback Loops
Utilize user feedback to continuously refine the spam filtering process. Allow users to report false positives and negatives, and incorporate their corrections into the training dataset.
3. Utilize Ensemble Learning
Combine multiple algorithms to enhance the robustness of your spam filter. Ensemble methods can help mitigate the weaknesses of individual models and improve overall performance.
Case Study: Successful Machine Learning Implementation in Spam Filtering
Numerous organizations have successfully integrated machine learning for email spam filtering. One notable example is a mid-sized tech firm that struggled with a high volume of spam emails. The company decided to implement a machine learning-based spam filtering solution. After a comprehensive analysis and model training phase, the organization noticed:
- A 90% reduction in spam emails reaching employee inboxes.
- A significant decrease in the time spent managing unwanted emails, leading to increased productivity.
- Enhanced employee satisfaction as important communications were less likely to be lost or overlooked.
Conclusion: Embracing the Future of Email Security
In an era where spam emails continue to pose a significant challenge to organizations, adopting machine learning for email spam filtering presents a valuable opportunity. By leveraging advanced algorithms and continuous data analysis, businesses can protect their communications from potential threats while improving operational efficiency.
For businesses looking to enhance their email security, the expertise provided by Spambrella.com offers comprehensive solutions in IT services and computer repair, as well as advanced security systems. Embrace the future of email communication with the power of machine learning and safeguard your organization against the ever-evolving threat of spam.